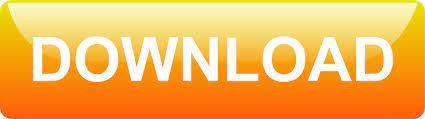
One of the common tools used to extract modes from climate data is principal component analysis (PCA), also known as empirical orthogonal functions (EOFs) ( von Storch and Zwiers 2001). Our understanding of the physics and impacts of this emergent behavior is therefore strongly impacted by the quality and purity of the modes we extract from data. 2017), and are also relevant for future forecasts ( Wu et al. The signal time series are often used to search for other phenomena driven by these large-scale patterns, such as heatwaves, droughts, and other temporary shifts in local climate ( Weisheimer et al. 2001) associated with these signals, and thus to better understand physical processes that generate climate variability and teleconnections. The spatial patterns and time series extracted from data are used to direct research into the physical mechanisms (e.g., Wanner et al.

Much climate research relies on extracting space and time signatures of large-scale climate processes such as El Niño–Southern Oscillation (ENSO). Finally, when applied to real climate data these alternative techniques extract a more coherent and less noisy global warming signal, as well as an El Niño signal with a clearer spectral peak in the time series, and more a physically plausible spatial pattern. We show that in the majority of simple cases PCA extracts modes that are not significantly better than a random guess. These techniques had a higher mean accuracy across modes in 60 out of 60 mixed synthetic climates, with SFA slightly outperforming DMD. We find that both alternative methods significantly outperform PCA in extracting local and global modes in the synthetic data. The datasets composed of these generated modes do not satisfy the assumptions of any pattern extraction method presented. We develop a Monte Carlo method to generate synthetic additive modes that mimic the properties of climate modes described in the literature. Here we analyze two modern pattern extraction methods, namely dynamical mode decomposition (DMD) and slow feature analysis (SFA), in comparison with PCA.
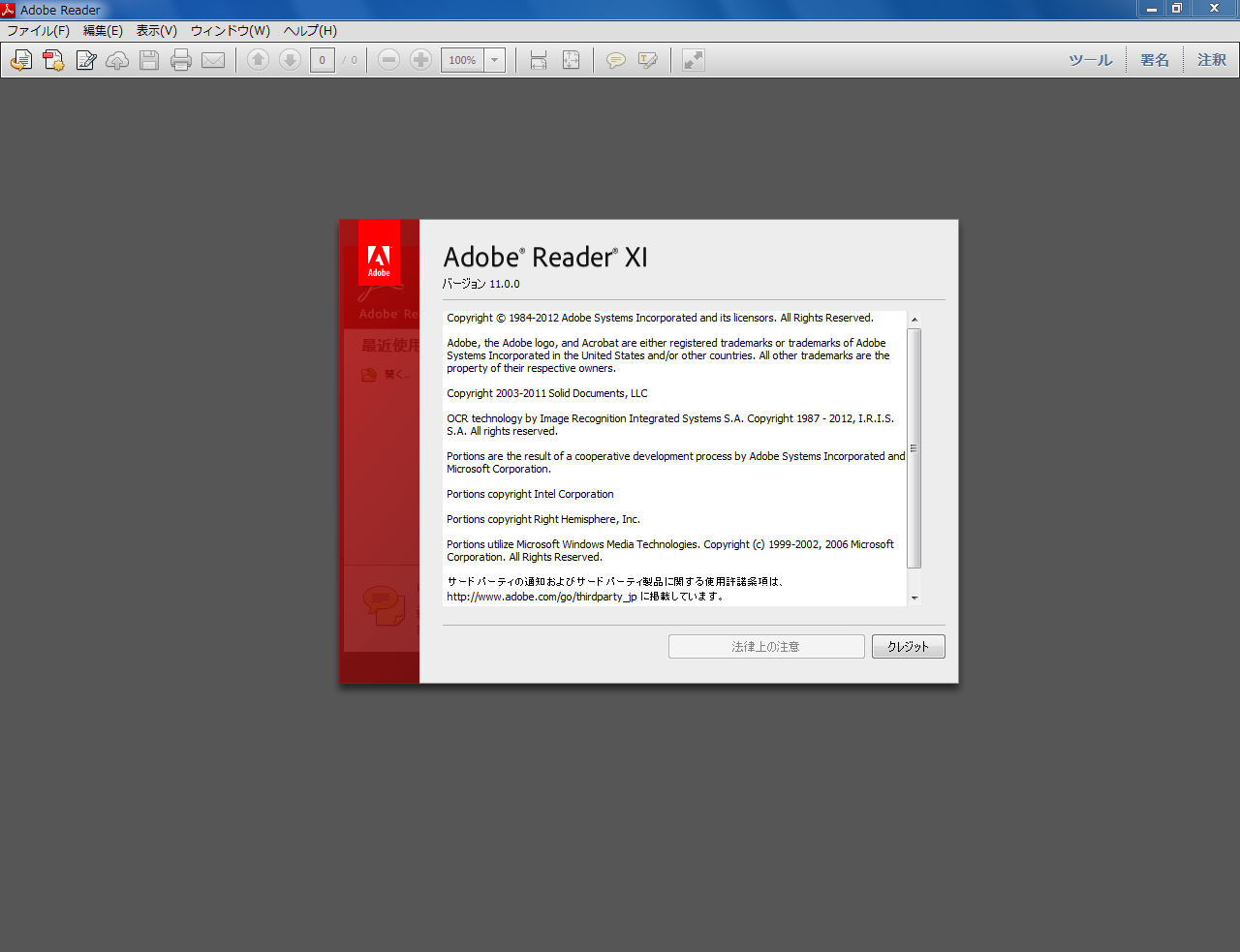
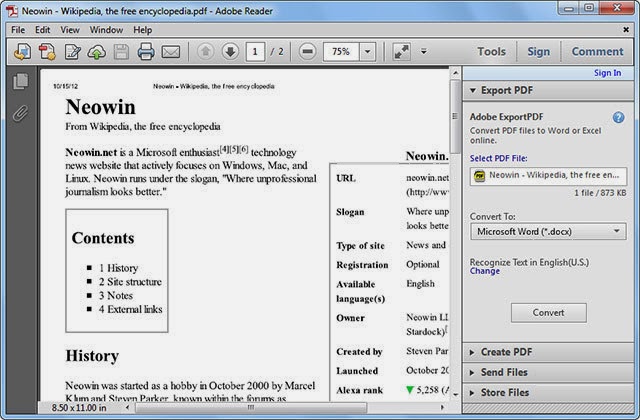
It has previously been shown that the standard technique of principal component analysis (PCA also known as empirical orthogonal functions) may extract patterns that are not physically meaningful. In this paper we develop a method to quantify the accuracy of different pattern extraction techniques for the additive space–time modes often assumed to be present in climate data.
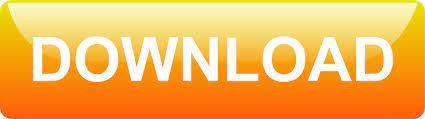